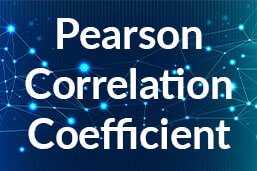
The best and most common method for measuring a linear correlation is calculating the Pearson correlation coefficient. This approach in statistics provides a significant formula in the field of experimental research. This article will account for the various types, how to calculate them, and the significant test. Furthermore, this guide will provide an in-depth understanding of using this method and give respective examples for visualization and clarity.
Definition: Pearson correlation coefficient
The Pearson correlation coefficient is an expressive statistic that measures the strength between diverse variables and how they relate. In simpler terms, it recaps the features of a dataset. The Pearson correlation coefficient is also known as:
- Bivariate correlation
- The correlation coefficient
- Pearson’s r
- (PPMCC) Pearson product-moment correlation coefficient
Its formula is as follows:
Types of Pearson correlation coefficients
The Pearson correlation coefficient is a digit between -1 and 1 that calculates the strength and course of the affiliation between two variables. The table below provides a vivid explanation.
Pearson correlation coefficient (r) | Correlation type | Interpretation | Example |
Between 0 and 1 | Positive correlation | A change in one variable triggers a change in the other in the same direction | Height and weight of a person: The taller a person gets, the heavier they weigh |
0 | No correlation | The variables are not affiliated | Cost of shoes and width of cars: The price of shoes will not influence the width of your cars and vice versa. |
Between 0 and -1 | Negative correlation | A change in one variable triggers a change in the other in the opposite direction | Elevation and temperature: The higher you go, the lower the temperature |
Positive correlation
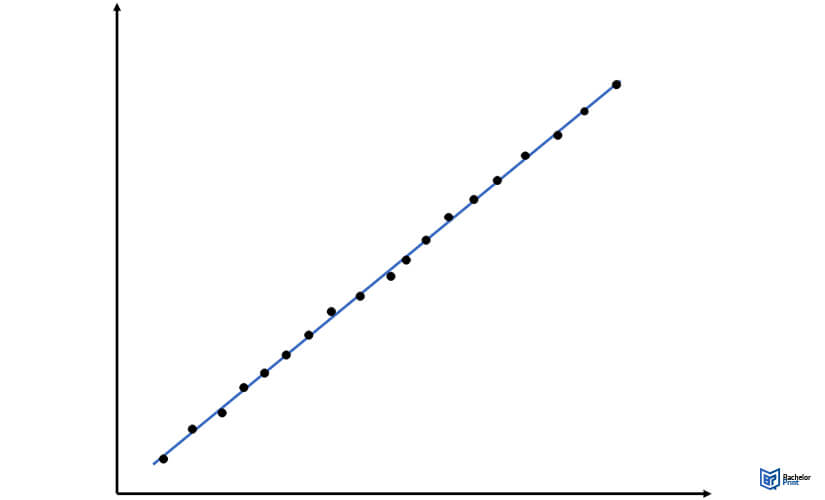
Negative correlation
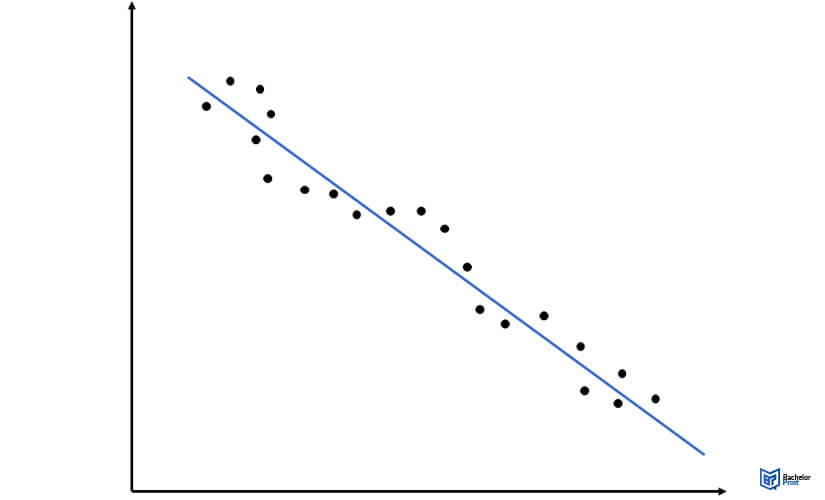
No correlation
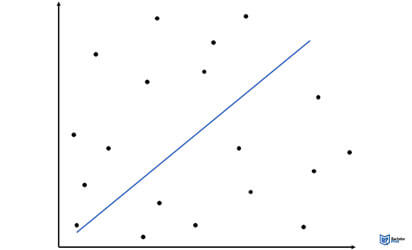
The effect size (relationship strength) interpretation may vary depending on the discipline. However, the following standard rules still apply.
Pearson correlation coefficient (r) value | Strength | Direction |
Higher than .5 | Strong | Positive |
.3 to .5 | Moderate | Positive |
0 to .3 | Weak | Positive |
0 | None | None |
0 to -.3 | Weak | Negative |
-.3 to -.5 | Moderate | Negative |
Below -.5 | Strong | Negative3 |
Besides descriptive statistics, the Pearson correlation coefficient can also be used for testing statistical hypotheses because it is an inferential statistic.
Visualizing the Pearson correlation coefficient
You can visualize Pearson’s r as a measure of how close the observations in experimental research are to a line of best fit. Also, it tells you whether the slope of the line of best fit is positive or negative.
Pearson correlation coefficient vs. Spearman’s rank correlation coefficients
Besides the Pearson correlation coefficient, another popular correlation coefficient is Spearman’s rank correlation coefficient.
It is a go-to method when at least one of the following characteristics is true:
- The variables are ordinal
- The variables are not distributed normally
- The data features outliers
- The variables have a non-linear or monotone relationship
Calculating the Pearson correlation coefficient
While the formula is easy to use, you can apply software tools like R or Excel to help you calculate the Pearson correlation coefficient.
Step 1: Calculating the sums of x and y
The first step is renaming the variables from weight and length to and
. Next, add up all the
and
values as indicated in the formula by the symbol
(take the sum of).
Step 2: Calculating x2 and y2 and the respective sums
Next, create two new columns containing the squares of the values in and
. Then, calculate the sums of the new columns.
Step 3: Calculating the cross product and its sum
Finally, create a column with the products of x and y and name it the cross product. Then, calculate the sum of the new column.
Step 4: Calculating Pearson correlation coefficient r
Use the formula above and the figures for each section to calculate the Pearson correlation coefficient.
Pearson correlation coefficient: Significance test
You can use the Pearson correlation coefficient to test if the relationship between two variables is significant.
For instance, if the Pearson correlation coefficient of the sample is r, then it is an estimate of rho, which is the correlation of the population. Therefore, determining the r and n (sample size) can help deduce if the rho is meaningfully different from 0.
-
Null hypothesis
-
Alternative hypothesis
You can use tools like the R or Strata software to test the hypothesis. Alternatively, you can follow these three steps:
Step 1: Calculating the t value
Calculating the t value is as easy as the following formula:
Step 2: Finding the critical value of t
You can find the t in a table that will need the following facts:
- The degree of freedom (df) (calculated using the formula: (
)
- Significance level α: Which is usually 0.05
- One-tailed or two-tailed: Two-tailed is the right option for correlations
Step 3: Comparing the t value to the critical value
Then, determine if the absolute t value is greater than the critical value. Note that “absolute” implies that you should disregard the minus sign if the t value is negative.
Step 4: Deciding whether to reject the null hypothesis
- If the t value is larger than
, the relationship is significant (p ˂ α). This information allows you to reject the null hypothesis and support the alternative hypothesis.
- If the t value is less than
, the relationship is insignificant (p ˃ α). This does not allow you to reject the null hypothesis or support the alternative hypothesis.
Pearson correlation coefficient in a thesis
The Pearson correlation coefficient usually comes up in the results section of an academic paper or thesis. Apply the rules below if you want to report in APA style:
- No need for a reference
- Italicize r
- Include a leading zero before the decimal point
- Provide two significant digits after the decimal point
- ✓ Free express delivery
- ✓ Individual embossing
- ✓ Selection of high-quality bindings
FAQs
It is calculated using the formula below:
You should use this method in inferential statistics or quantitative statistics. You can also use it to test correlations between two variants.
It helps test the relationship between two variants. It also helps determine the course of change if either variant is altered.
It isn’t easy to calculate. However, if you master the formula, you should be okay.