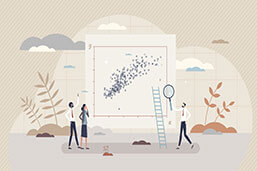
In mathematical and scientific studies, statistical significance serves as a tool to help researchers assess whether the outcome of a study is a result of chance or depicts a true effect. For this, the p-value is commonly used to reject or confirm the null hypothesis. In the case of rejection, it can be determined that there is no effect between variables. If it is confirmed, it can be determined that a true effect or relationship is present, meaning the results are statistically significant. Learn more about statistical significance in this article.
Definition: Statistical significanc
Statistical significance is a claim or determination made by a researcher that a group of observed data results from a particular cause instead of the product of chance or coincidence. The statistical significance can be described as strong or weak. When used in statistics, this concept is often expressed in terms of a p-value, which is a probability measurement used in observing data, given that the null hypothesis is true. The null hypothesis usually assumes that there is no relationship or effect between variables. If the p-value is less than the threshold (usually set at 0.05), the results are deemed statistically significant. This assesses that the observed data has a highly unlikely chance of occurring if the null hypothesis were true, thus providing evidence against the null hypothesis and in favor of an alternative hypothesis.
Testing for statistical significance
Testing for statistical significance is essential in quantitative research. Researchers conducting quantitative studies analyze their observed data through hypothesis testing. Therefore, statistical significance testing is the formal way of evaluating the correlation between variables or sets of data. The following presents guidelines for testing statistical significance:
Null and alternative hypotheses
The first step is categorizing the research predictions into null and alternative hypotheses. Hypothesis testing always begins by assuming that the null hypothesis is correct or justified. After assuming the null hypothesis is accurate, you can use hypothesis testing to assess the probability of obtaining your research results under this assumption. The outcome of your test will help you determine whether to reject or accept your null hypothesis.
Test statistics and proportional values (p-values)
All statistical tests produce a test statistic and p-value.
- A test statistic indicates how closely your observed data matches the formulated null hypothesis.
- The p-value indicates the probability of getting the study outcome if the null premise is correct.
A low p-value means that a result is not easily explainable by chance alone; hence the null hypothesis can be rejected. In contrast, a large p-value means that the result is explainable by chance alone, so you can retain the null hypothesis. Therefore, the p-value determines the statistical significance.
Statistical significance and significance level
The significance level is a value set by a researcher before the experiment as the brink for statistical significance. The significance level is the extreme jeopardy of making a false optimistic inference that you are prepared to take. The significance level measures the strength of the evidence that must be present in your sample before you decide to reject or accept the null hypothesis.
A hypothesis test always ends by comparing the p value to the significance level. This helps you determine whether to retain or castoff the null hypothesis.
- If the p-value is greater than the significance level, it means that the null supposition is not disproved and the results of the study are not statistically noteworthy
- If the proportional value is smaller than the significance level, the outcomes are statistically significant and are construed as rebutting the null hypothesis.
Most researchers set the significance level at 5%. A 0.05 significance level indicates a 5% risk of concluding that a difference exists when no difference exists. It is worth mentioning that hypothesis testing only shows you whether to castoff or maintain the null hypothesis in favour of the alternate hypothesis.
The problem with statistical significance
The main problem with statistical significance is that it is oftentimes categorized as statistically significant or not based on conformist thresholds that lack theoretical backup. This implies that a slight decrease in the p-value can alter the findings from insignificant to significant, even if there is no noteworthy change in the effect.
Furthermore, statistical significance can be misleading when used independently. This is because the sample size affects it. For instance, in large samples, the probability of obtaining statistically significant results is high even whether the effects are minimal or not noteworthy in real word contexts.
- ✓ Free express delivery
- ✓ Individual embossing
- ✓ Selection of high-quality bindings
Types of significance in research
Apart from statistical significance, you can use the following to predict research outcomes:
- Practical significance determines if the research results are vital enough to be valuable in the real world. Therefore, it is indicated by the study’s effect size.
FAQs
Statistical significance is the claim that a set of observed information or data is not the result of coincidence but can be credited to a particular cause.
Statistical significance measures the likelihood of a study’s null hypothesis being correct, likened to the acceptable level of ambiguity concerning the correct answer.
A hypothesis test always ends by comparing the p value to the significance level.
The p value measures the likelihood that an observed difference could have occurred by coincidence. It determines a result’s statistical significance.