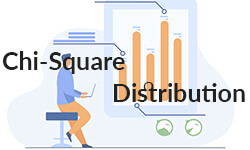
The chi-square distribution is an essential concept within statistics, frequently used as the essence for statistical tests, such as the chi-square test of independence and the chi-square goodness-of-fit test. In general, the chi-square distribution poses a framework in inferential statistics and hypothesis testing, particularly in relation to assessing statistical significance and dealing with categorical data.
Definition: Chi-square distribution
The chi-square distribution is a constant hypothetical dispersal of values for a population. It is commonly applied in statistical tests. The parameter k, which denotes the degrees of freedom, governs the outline of a chi-square distribution. The chi-square distribution applies to theoretical distributions. In contrast, normal and Poisson distributions apply in real-world distributions.
Chi-square distribution vs. standard normal distribution
The chi-square distribution is closely related to the standard regular dispersal; hence its application in hypothesis testing. The standard normal distribution is a normal distribution with a mean and variance of zero and one, respectively.
Evaluating the k degrees of freedom
Generally, if you are ample from k autonomous average nominal distributions and square and quantify their values, you will yield a chi-square distribution in the existence of k degrees of freedom.
Therefore:
X2k= (Z2)2+XXX+(Zk)2
Chi-square distribution formula
Chi-square assessments are types of hypothesis tests whose test statistics tail a chi-square distribution in the null proposition. The most common chi-square test is Pearson’s chi-square assessment, which was also the first to be discovered.
The table explains Pearson’s chi-square test figure:
where
The test measurement of a sampled population usually follows a chi-square distribution if the null hypothesis is correct. This applies where you sample a population severally and find the Pearson’s test statistic for every trial.
Chi-square distribution shape
Graphs of the chi-square probability compactness function illustrate how the chi-square distribution alters as k increases. A concentration function defines a continuous prospect dispersal.
K = 1 or K = 2
The outline of a chi-square distribution alters depending on the value of k. When k is ½, the shape curves back into a “J.” This implies a high probability of the X2 being close to zero.
K greater than 2
When the parameter is better than two, the chi-square distribution appears hump-shaped. This implies that it starts out low followed by an increase, then a decrease. This denotes a low probability of X2 being close to zero. In contrast, when the parameter (k) is slightly more than two, the chi distribution will be longer on the right peak side than on the left.
The chi distribution tends to resemble the normal distribution as k increases. In some instances (when k is 90 or more) the normal distribution applies as an estimate of a chi-square distribution.
Printing Your Thesis With BachelorPrint
- High-quality bindings with customizable embossing
- 3D live preview to check your work before ordering
- Free express delivery
Configure your binding now!
Properties of a chi-square distribution
Chi-square distribution usually has some standard properties. Here are its properties:
Property | Symbol |
Type (Discrete/ continuous) | Continuous |
Variance | 2k |
Mean | K |
Standard deviation | Square root of 2k |
Mode | K – 2 (if k less than 2) |
Range | Zero to infinity |
Symmetry | Rightly-skewed, increasing as k increases |
Chi-square distribution example
The chi-square distribution is applied in many statistical and theoretical tests. Here are the most frequent tests it is applied on:
Pearson’s chi-square test
This is a statistical test for definite statistics used to determine the significance of the difference between the data and your expectations.
There are two categories of Pearson’s chi-square tests:
- Chi-square goodness of fit test
- Chi-square test of independence
Population variance inferences
The chi-square distribution comes in handy when making extrapolations about standard deviation or variance. It helps with hypothesis testing to determine if the population variance equates to a specific value or to calculate its confidence intervals.
F distribution
The chi-square distribution helps with defining the incidence distribution, especially in ANOVAs.
Non-central chi-square distribution
This is an overall kind of chi-square distribution used in some forms of power analyses. It features an extra lambda and non-central parameter, which changes its shape. Its peak shifts to the right and increases as the variance grows. The lambda parameter defines the mean figure of the normal dispersal.
FAQs
The curve shifts from downward to hump-shaped. The more the k increases, the more right-skewed it gets.
The chi-square distribution is a constant probability dispersal commonly applied in hypothesis testing.
There are two types. They are:
- the chi-square test of good fit
- the chi-square freedom test
The chi-square distribution is used to define the quantity of a squared random variable.