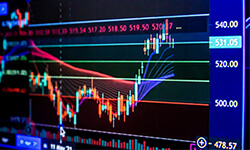
The Chi-square distribution table is a vital tool in the field of statistics, often used for hypothesis testing and goodness-of-fit tests. It provides critical values that help statisticians make inferences about population parameters based on sample data.
When testing a hypothesis, you can use a chi-square distribution table to calculate the confidence interval for certain parameters and investigate their statistical significance.
Definition: Chi-square distribution table
In statistics, a chi-square table lists the critical values of a chi-square distribution for different levels of significance and degrees of freedom. The degrees of freedom are proportionate to the number of variables under analysis. On the other hand, significance levels show the confidence intervals between observed and expected parameters.
To calculate the chi-square values, take the difference between observed and expected values, square the results, and divide the outcome by the expected value. If the result exceeds the expected value, it means there is a significant difference between your sample data and the null hypothesis.
When to use a chi-square distribution table
Chi-square critical values and the chi-square distribution table are statistical tools used to test hypotheses. To conduct a chi-square test, you will calculate a chi-square statistic that shows the discrepancy between observed and predicted data. You will then analyze the difference to identify its significance to the hypothesis.
The chi-square distribution table can help you find the critical values of a chi-square distribution. It contains a list of critical values for chi-square tests with varying degrees of freedom and significance levels. The table allows you to compare the differences between observed data against critical values to conclude your hypothesis.
The chi-square distribution table
Chi-square distribution tables based on right-tail probabilities list the critical values of a chi-square distribution for a defined level of significance and degrees of freedom. Here is an example of a right-tailed chi-square distribution table:
Using the symmetry of the chi-square distribution table, you can find the left-tail probabilities of the data. By subtracting one from the right-tail probability values of the table, you can get the left-tail probability values.
For instance, if the right-tail probability for a given critical value is 0.10, the left-tail probability value would be 1 – 0.10 = 0.90. As a result, there is a 90% chance of getting a chi-square value lower than or equal to the critical value.
Note: Some chi-square distribution tables provide lists for both right-tail and left-tail probabilities for each critical value.
Directions to using the chi-square distribution table
You may need to consult a chi-square distribution table to find the critical values or p-values for a chi-square test. Here are the three steps you should follow when using the table:
Degrees of freedom
In a chi-square distribution table, degrees of freedom (df) refer to the number of independent variables available for testing a hypothesis. In the table, you will find the degrees of freedom in the first column.
There are several types of chi-square distributions. Each has its own equation for calculating the degrees of freedom. The common ones include:
- Chi-square goodness of fit test: used to test whether a categorical variable fits a theoretical distribution. i.e. df = the number of categories minus 1.
- Chi-square test for independence: used to test whether two categorical variables are independent. i.e. df = the number of rows minus 1 multiplied by the number of columns minus 1.
- Chi-square test for homogeneity: used to test whether there is a difference in proportionality between several groups of variables. df= the number of groups minus 1.
Significance level
You can find the significance levels of a chi-square distribution table on the top row. The significance level is often used in conjunction with the p-value to determine the significance of the results in a test. It is a measure of the strength of evidence against the null hypothesis.
A commonly used significance level in statistical analysis is 0.01, which indicates that there is a 1% chance of rejecting the null hypothesis when it is true. It implies that if the calculated p-value is less than 0.01, you should disregard the null hypothesis at a 1% significance level.
When testing hypotheses, you can encounter two types of errors. Type I errors, denoted by the significance level (α), occur when you reject a null hypothesis when it is true. Type II errors (β) occur when one fails to reject a null hypothesis when it is false.
It is essential to consider the potential consequences of committing each type of error and choose an appropriate balance between the two based on the context of the research question and available resources.
Critical value
The critical values in a chi-square distribution table are at the intersections of rows and columns for a given significance level and the corresponding degrees of freedom. They are threshold values used in testing hypotheses to determine whether to reject a null hypothesis.
If your test statistic is higher than the critical value in the chi-square table, you can disapprove the null hypothesis and vice versa.
Printing Your Thesis With BachelorPrint
- High-quality bindings with customizable embossing
- 3D live preview to check your work before ordering
- Free express delivery
Configure your binding now!
Left-tailed & two-tailed chi-square distribution tables
The decision to use a right-tailed table or a left-tailed table depends on the direction of the statistical test.
If you suspect that the observed parameter will be greater than the assumed parameters of the null hypothesis, then you should use a two-tailed table. Consequently, if you expect a lower-value outcome than the assumed results, use a left-tailed table.
The most commonly used left-tailed test is the t-test. A left-tailed test determines whether the statistic is significantly less than the expected outcome.
In a two-tailed test, you examine the area under both tails of a given distribution curve. The most common two-tailed test is the two-sample t-test. It shows whether there is a significant difference between the means of a sample and a population.
FAQs
A chi-square distribution table helps determine the probability of obtaining a particular chi-square value in a hypothesis test.
It is a reference point that shows the probability values associated with different levels of significance and degrees of freedom for the chi-square distribution.
Degrees of freedom (df) refer to the number of independent variables in a sample. In a chi-square distribution table, df is the total number of categorical variables minus one.
You use a one-tailed test to examine for a specific direction of association between two variables. Alternately, a two-tailed test investigates any association between two variables, whether positive or negative.