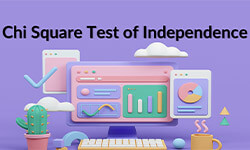
The chi-square test of independence is a statistical test used to determine whether two categorical variables are associated or independent. A way to assess the independence or dependence of variables is to use a contingency table, allowing you to compare the expected frequencies with the observed ones. In the realm of statistics, the chi-square test serves as a valuable tool across fields, such as marketing, social science, and medical research.
Definition: Chi-square test of independence
The chi-square test of independence is a statistical test used to determine the association between two categorical variables. The chi-square test of independence, also known as Pearson’s chi-square test, is a widely used nonparametric test because it does not rely on the assumptions of parametric tests, particularly the assumption of a normal distribution.
The chi-square test of independence is calculated by comparing the observed frequencies of categories in a contingency table with the frequencies that would be expected if the variables were independent. The components needed for the test are the observed frequencies, expected frequencies, and degrees of freedom.
Contingency tables
Contingency tables summarize and display the relationship between two categorical variables in the chi square test of independence. They are cross-tabulation tables, two-way frequency tables, or crosstabs.
They are useful for analyzing the relationship between two categorical variables, and they can be used as the basis for statistical tests such as the chi square test of independence.
The chi-square test of independence hypotheses
The chi-square test of independence is used to test whether the observed frequencies of the categories in a contingency table differ significantly from those expected if the variables were independent.
The hypotheses for the chi-square goodness of fit test could be:
Expected values
Expected values in the context of the chi square test of independence refer to the frequencies that would be expected if the two categorical variables were independent.
The formula for calculating the expected frequency for each cell of a contingency table is:
When is the chi square test of independence used?
The chi square test of independence can be used when certain criteria and circumstances are met:
- The variables under investigation are categorical or nominal
- The variables are independent of each other
- The expected frequency count for each cell in a contingency table is at least 5
If these criteria are met, the chi square test of independence can be used to test whether there is a significant association between the two categorical variables.
Calculating the test statistic of the chi-square of independence
The formula for calculating the test statistic of the chi square test of independence is:
where
The chi-square test statistic measures the difference between the observed and expected frequencies in a contingency table.
To calculate the test statistic for the chi square test of independence, follow these five steps:
- Create a contingency table with the observed frequencies for the two categorical variables.
- Calculate the expected frequencies for each cell in the contingency table.
- Calculate the difference between each cell’s observed and expected frequencies, and square the difference.
- Divide the squared difference by the expected frequency for each cell.
- Sum the values obtained in step 4 to get the chi-square test statistic.
1. Table of frequencies
To conduct the chi square test of independence, the first step is to establish a contingency table containing the counts or frequencies of each category of one variable for each category of the other variable.
2. Calculating O – E
This step of chi square test of independence helps to quantify the extent to which the observed frequencies differ from what would be expected under the assumption of independence between the two variables.
To calculate O – E, an additional column is added to the contingency table to represent the difference between the observed and expected frequencies for each cell.
Using the previous example of the medical intervention and patient outcome, the contingency table with added columns would be:
3. Calculating (O – E)²
To calculate (O – E)², another column is added to the contingency table. This third step of calculating the chi square test of independence assesses the squared difference between each cell frequencies of observed and expected values.
Using the same example of the medical intervention and patient outcome, the contingency table with the additional columns would be:
4. Calculating (O – E)²/ E
To calculate , an additional column is added to the contingency table to represent the result of dividing the squared difference between the observed frequency and the expected frequency by the expected frequency for each cell.
This step scales the contribution of each cell to the overall chi-square test statistic.
5. Calculating X²
The last step in the chi square test of independence is to sum the values in the column to obtain the overall chi-square test statistic. This test statistic measures the degree of association between the two categorical variables.
Continuing with the same example of the medical intervention and patient outcome in our chi square test of independence, we can sum the values in the column as follows:
Performing the chi square test of independence
When performing the chi square test of independence, a large value of the chi-square test statistic indicates that the observed frequencies in the contingency table are significantly different from the expected frequencies under the assumption of independence between the two categorical variables.
The six steps to perform the chi square test of independence are:
1. State the null and alternative hypotheses
2. Create a contingency table
3. Calculate the expected frequencies
4. Calculate the chi-square statistic using the formula:
5. Determine the degrees of freedom and p-value
6. Interpret the results association.
1. Calculating the expected frequencies
The first step in using the chi square test of independence is to calculate the expected frequencies for each cell in the contingency table. The formula for calculating the expected frequency for a cell is:
2. Calculating the chi-square
The second step of the chi square test of independence is to calculate the test statistic (χ²) using the formula: , where O is the observed frequency and E is the expected frequency.
where
3. The critical chi-square value
The critical chi-square value can be found in a chi-square distribution table or software, based on the chosen level of significance and the degrees of freedom (df). The formula for degrees of freedom for the chi square test of independence is:
where
The significance level is typically set at 0.05 or 0.01.
4. Comparing the chi-square value to the critical value
The next step in the chi square test of independence is to compare the calculated chi-square test statistic to the critical value obtained from the chi-square distribution table or software. If the calculated chi-square test statistic is greater than the critical value, the null hypothesis is rejected and it is concluded that there is a significant association between the two categorical variables.
5. Should the null hypothesis be rejected?
If the calculated chi-square test statistic is greater than the critical value, the null hypothesis is rejected, indicating a significant association between the two categorical variables. If the calculated chi-square test statistic is less than or equal to the critical value, the null hypothesis is not rejected, indicating no significant association between the two categorical variables.
Practice questions for the chi-square test of independence
How much knowledge do you have regarding the chi-square test of independence? The ideal and convenient method to find out how much you know is by asking yourself some practice questions for the chi-square test of independence. Therefore, the downloadable document below will explore some practice questions for the chi-square test of independence and their answers.
Chi-square test of independence vs. other tests
Apart from chi-square test of independence, some other tests in other scenarios include:
Test | When to use it |
Chi-square goodness of fit | When there is only one categorical variable and we want to test whether the observed frequencies fit a known or expected distribution. |
Fisher’s exact test | When the sample size is small (typically less than 20) and the expected frequency for one or more cells is less than 5. |
McNemar’s test | When the data are paired or matched, such as in a before-and-after study or a case-control study. |
G test | When the sample size is small or the expected frequency for one or more cells is less than 5, and when the Chi-square test is not appropriate due to its assumptions. |
Printing Your Thesis With BachelorPrint
- High-quality bindings with customizable embossing
- 3D live preview to check your work before ordering
- Free express delivery
Configure your binding now!
FAQs
To perform a chi square test of independence in R, you can use the chisq.test() function, specifying the two categorical variables you want to test for independence. The function returns the test statistic, degrees of freedom, and p-value for the test.
A chi square test of independence is a statistical method used to determine if there is a significant association between two categorical variables.
To perform a chi square test of independence, the researcher creates a contingency table and calculates the chi-square statistic by comparing observed and expected frequencies.
The p-value is then calculated to determine if the null hypothesis is rejected or accepted in the chi square test of independence.
If the p-value is less than 0.05, the two variables have a significant association. If the p-value exceeds 0.05, there is no significant association. Another way, is to calculate the effect size, which can also determine the strength of the association.