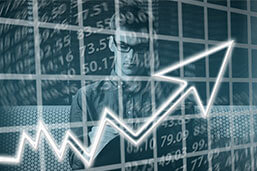
Statistical power, a critical concept in statistical analysis, refers to the likelihood that a study will detect an effect when there is an effect to be detected. This measure plays a vital role in academic writing, helping scholars to determine the adequacy of their sample size and the robustness of their findings. In the realm of statistics, understanding and correctly applying statistical power can vastly improve the validity and reliability of research outcomes.
Definition: Statistical power
Statistical power refers to the probability of a hypothesis test identifying a true effect if one exists. A true effect in statistics implies a non-zero, real association between a set of variables in the target population.
- High power is a significant likelihood of a test uncovering a true effect
- Low power implies that a statistical test has a slim chance of sensing a true effect.
Importance of statistical power
Statistical power is essential to derive accurate findings from samples selected in a population. Hypothesis testing begins with a null and an alternative hypothesis proposed to contradict the null hypothesis.
Analysis of study results is prone to two common errors:
Type I error
Disregarding the null hypothesis of zero effect when it is true.
Type II error
Failing to reject the null hypothesis of no effect though it is false.
A high statistical power such as 80% reduces the chances of a Type II error. Low power tests may completely fail to detect true effects. On the other hand, too much power leads to very sensitive tests.
Statistical power – Power analysis
Power analysis is a method used to estimate the smallest sample in a study. If you have three of these components, you can estimate the fourth. There are four primary parts of power analysis:
Significance level (alpha) | The highest risk you are willing to consider in disapproving a null hypothesis, usually maintained at 5%. |
Statistical power | The probability that a test will identify the presence of an effect if it exists, starting from 80% and above. |
Sample size | The base number of observations required to note an effect of a certain level with a specified power level. |
Expected effect size | A conventional way of illustrating the strength of the desired outcome of the study. |
You can conduct a power analysis before a study, the recommended significance level is 5%, while the desired power level is set to 80%.
Sample size
A small sample (up to 30 units) typically has low power, and expanding the sample size increases the power but only to a limited extent.
The designated research design is also affected by power and sample size as follows:
Within-subjects design | Every participant in a study is tested in all conditions, which prevents individual variations from affecting the outcome of various conditions. |
Between-subjects design | Each study member is subjected to a single condition. Since every condition is assigned to a different participant, individual contrasts may affect the outcome. |
The within-subjects framework is more capable; therefore, few subjects are needed.
Significance level
The significance level implies the likelihood of the Type I error and is often maintained at 5%. Your outcomes must have a less than 5% chance of happening to be regarded as statistically significant in a null hypothesis.
Researchers account for their risk tolerance in making false positives and negatives to regulate the likelihood of making Type I and II errors.
Effect size
Studies with high power can identify large and medium effects, while low-powered studies can only detect large effects.
In fields such as engineering, using low-powered simulations as the norm may result in an exaggerated estimation of true effects.
Statistical power – Other influencing factors
Besides the main elements of statistical power, researchers should consider other factors in estimating power. They include:
Variability
Data groups with large variations decrease the sensitivity of a test while a group with smaller variations increases sensitivity. A defined population with demographic markers can reduce the variation of the main variable and boost power.
Measurement error
This is the difference between a true value and the identified value of a measurable object. The two types of measurement error are:
Error type | Definition | Example |
Random errors | They occur because of chance since they are unpredictable. | Attitudes and moods tend to fluctuate during a study which may affect the quality of measurements. |
Systematic errors | Errors that are predictable as they are technical in nature. | Poorly constructed research questions often cause biased outcomes. |
Increasing the statistical power
There are several ways of increasing statistical power, as discussed below:
Increasing the effect size
Researchers can adjust the independent variable upwards, for example, expanding the social circle to measure marginal improvement in happiness.
Increasing the sample size
Additionally, there may be room to increase the sample size using sample size satiates as a basis.
Increasing the significance level
Though this increases sensitivity, it also creates a higher probability of a Type II error.
Reducing measurement errors
Using high-quality, well-calibrated machines reduces measurement errors. Researchers may also employ multiple instruments and methods.
Using a one-tailed test instead of a two-tailed test
One-tailed tests have a higher power in t and z tests. However, one-tailed tests are limited to cases with a good reason to anticipate an effect in a particular direction. A two-tailed test is applicable in testing effects in any direction.
Printing Your Thesis With BachelorPrint
- High-quality bindings with customizable embossing
- 3D live preview to check your work before ordering
- Free express delivery
Configure your binding now!
FAQs
Statistical power is the probability that a statistical test will detect a true effect if one is present, and a high statistical power rejects the null hypothesis.
The null hypothesis postulates that there is no real difference between populations, and statistical power tests aim to reject the null hypothesis.
Researchers prefer high statistical power for various reasons. They can improve statistical power by altering variables such as confidence level and adjusting the sample size.
Power analysis refers to calculations to find the minimum sample for a study. The optimum sample size helps to increase the accuracy of a case study.