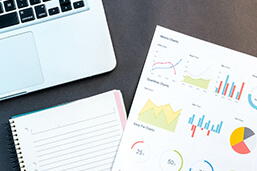
Variance, a fundamental concept in statistics, is derived by computing the average of squared deviations from the mean, providing an indication of the dispersion within your data set. A greater variance relative to the mean signifies a higher degree of distribution in the data set. The following article will give you more profound insights on the topic and illustrate it with various formulas.
Definition: Variance
The variance, also called mean square deviation, is a measure of variability, showing the dispersion of data around the mean. It is defined as σ2, the square of the standard deviation. The larger the spread of the data is, the more the variance differs from the mean. However, since the mean square deviation is squared, the values are not intuitive to interpret and the standard deviation is of more use to non-experienced researchers.
Step-by-step calculation
Typically, the program you use for your statistical study will automatically calculate the mean square deviation. However, you may also perform a manual calculation to better comprehend how the formula functions.
When determining the mean square deviation manually, there are five key phases:
Step 1: Determine the mean
To find the mean, sum up all values x, and divide them by the number of values n.
Step 2: Deviation from the mean
To determine the deviations from the mean, subtract the mean from each score.
Step 3: Square each deviation
Add up each deviation from the mean that produces a positive number.
Step 4: Sum up the squares
The squared deviations are totaled and called the sum of squares.
Step 5: Divide the sum of squares by
(n – 1) or N
Divide the sum of the squares by (n-1) for a sample or N for a population.
or
Population vs. sample variance
To calculate the population variance σ2, you need to gather data from every single person in your population. This can be an entire class, a school, a company, etc., since “population” does not always refer to the entirety of humans in the world. It is calculated by dividing the sum by the number of individuals in the entire population.
It is still more likely that your study is conducted by using a sample, a selection of subjects from the population, to gather your data. The sample variance s2 is used to make estimations towards the population mean square deviation. It is calculated by dividing the sum by the number of individuals in the sample minus one.
Grouped vs. ungrouped data
Grouped data is usually used with continuous variables, those who are presented in fractions and decimals and where repeated values rarely happen. An example for continuous data would be length or height, since you can measure these extremely exact in millimeters, decreasing the probability of two people having the exact same height. Age is also a continuous variable because in a sample, you rarely have more than two or three people with the exact same age.
The formulas in the former paragraph are those used for ungrouped data. Grouped data is always presented in intervals, which need to be considered in the calculation.
or
In this case, m is the middle value of each interval (calculated by adding the upper border to the lower one before dividing the sum by 2) and f is the frequency of the interval, meaning the number of values the interval contains (in an exemplary interval reaching from 45-55 containing 4 values, f=4 and not 10, which would be the width). Here, n is the number of subjects in your sample. The mean of grouped data is calculated using the following formula:
Weighted variance
The weighted variance is calculated, when each value of the dataset is assigned a weight, depending on how often each value should be counted in the final equation. To calculate the weighted variance, you first need to calculate the weighted mean (sometimes also referred to as µ*), using the following formula:
In the final formula, the weight is multiplied with the square and the whole sum is then divided by the sum of weights.
Standard deviation
The standard deviation σ is calculated by extracting the square root from the variance. Therefore, the standard deviation also has far smaller quantities in units (e.g., meters, while the variance would be square meters). This makes it more intuitive to grasp. Generally, the standard deviation, which can also be called mean deviation, is the average distance between a value of the dataset and the mean.
Covariance
While the variance compares each value to the mean, the covariance compares it to another variable. This means that the covariance only exists in studies with at least two different variables (e.g., height and age). Therefore, you subtract the mean of each variable from the individual values before multiplying it with the same difference of the other variable before summing up the results.
or
Printing Your Thesis With BachelorPrint
- High-quality bindings with customizable embossing
- 3D live preview to check your work before ordering
- Free express delivery
Configure your binding now!
Usage
The mean square deviation is significant for two fundamental reasons:
- Mean square deviation is susceptible to parametric statistical tests.
- You can evaluate group differences by comparing a sample mean square deviations.
1. Homogeneity of variance in statistical tests
Prior to conducting parametric testing, variation must be considered. Also known as homogeneity of mean square deviation or homoscedasticity, these tests require identical or comparable mean square deviations when comparing various samples.
Test results are skewed and biased due to unequal variances between samples. Non-parametric tests are better suited if sample variances are uneven.
2. Using variance to assess group differences
The sample mean square deviation is used in statistical tests to evaluate group differences, such as variance tests and the analysis of variance (ANOVA). They evaluate whether the populations they represent are distinct from one another using the mean square deviations of the samples.
3. An ANOVA is used to evaluate group differences
The basic goal of an ANOVA is to evaluate variances within and across groups to determine whether group differences or individual differences can better account for the results.
The groups are probably different due to your treatment if the between-group mean square deviation is higher than the within-group mean square deviation. If not, the outcomes could originate from the sample members’ unique differences.
FAQs
- Range: the difference between the highest and lowest value
- Interquartile range: the range of a distribution’s middle half
- Standard deviation: the typical departure from the mean
- Variance: squared mean deviations are averaged out
The standard deviation is the average-squared deviation from the mean.
Both metrics capture distributional variability, although they use different measurement units. The units used to indicate standard deviation are the same as the values’ original ones, such as minutes or meters.
The sample discrepancy is used by statistical tests to evaluate population group differences, such as variance and the analysis of variance (ANOVA).
They determine whether the populations they represent significantly differ from one another using the sample variances.
Homoscedasticity, also known as homogeneity of the mean square deviation, is the presumption that variations in the groups being compared are equivalent or similar.
Because parametric statistical tests are sensitive to any differences, this is a crucial presumption. Results from tests are skewed and biased when the sample mean square deviation is uneven.