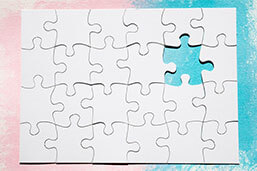
Even well-designed and controlled research studies may come across the issue of missing data. Missing values reduces a study’s statistical power and causes erroneous estimates and inaccurate findings. Missing data arise in the form of nonresponse in surveys, unrecorded observations, or attrition in longitudinal studies. Addressing missing data appropriately is crucial and can be done through several methods. This article discusses missing data concerns, types, and ways of dealing with them.
Definition: Missing data
In statistics, missing data refers to the absence of values for certain variables or observations in a dataset. This can happen due to a participant not responding to a survey question, a failure in data collection, or data being lost or overlooked during analysis. Missing data can challenge analyses, as standard statistical methods often assume complete data for all variables. Depending on the degree and type of the missing data, bias, reduction of the statistical power, and impact on the validity of the results may be a byproduct. Therefore, it is crucial to handle missing data appropriately to ensure reliable conclusions.
Types of missing data
Missing data are errors because they do not represent the actual values of what was intended to be measured.
Consideration of the reason for the missing values is essential, as it enables you to establish the type of missing values and the necessary course of action.
Missing values autumn into three categories:
MCAR |
Missing completely at random (MCAR) occurs when the probability of missing data is unrelated to the expected value or observed responses. MCAR is an ideal but impractical assumption for many anaesthesia studies. MCAR data are missing by design due to instrument failure or because samples are lost in transit or are technically unacceptable. MCAR data ensures unbiased analysis. The design may lose power, but missing value doesn't influence estimated parameters. |
MAR | MAR is a better assumption for anesthetic studies. MAR data are missing when the probability of missing replies relies on the observed responses but not the expected missing values. We may think MAR isn't a concern because randomness isn't biased. Missing data can't be ignored under MAR. If a dropout variable is MAR, the probability of a dropout in each case is conditionally independent of the variable obtained currently and expected to be obtained in the future, given the history of the obtained variable before that case. |
MNAR | If data characters don't meet MCAR or MAR, they're missing, not at random (MNAR). MNAR data is problematic. Modeling the missing data is the only approach to getting unbiased parameter estimates. The model is then used to estimate missing values. |
How to prevent missing data
Common causes of missing values include attrition, non-response, and poorly constructed study techniques. While planning a study, it is advisable to make it simple for participants to contribute data.
Here are tips to minimize missing values:
✓ Limit follow-ups
✓ Minimize data collected
✓ Make forms user-friendly
✓ Incorporate methods of data validation.
✓ Give rewards
How to deal with missing data
Typically, you have the choice of accepting, eliminating, or reconstructing missing values to organise your data.
Determine how to handle each instance of missing values depending on your evaluation of the missing value’s cause:
- Are these missing data due to random or non-random causes?
- Are missing data zero or null?
- Was the query or measurement ill-conceived?
If your information is MCAR or MAR, it can be accepted or left unchanged. However, MNAR data may necessitate a more intricate approach.
Missing data: Acceptance
Accepting missing data is the most prudent course; leave these cells blank. This is best for MCAR or MAR values. When you have a small sample, save as much data as possible to maintain statistical power.
To make your dataset consistent, recode any missing values as “N/A.” These steps let you preserve as much research data as possible without alterations.
- ✓ 3D live preview of your individual configuration
- ✓ Free express delivery for every single purchase
- ✓ Top-notch bindings with customised embossing
Missing data: Deletion
Listwise or pairwise deletion can be used to eliminate missing values from analyses.
Listwise deletion
Listwise deletion eliminates all cases (participants) with missing data for any variable. You’ll have the entire participant data. This strategy may result in a smaller, biased sample. If data are lacking from some variables or measurements, those who offer them may differ from those who don’t.
Your sample may not be representative of the population, making it biased.
Pairwise deletion
Pairwise deletion removes data only if a needed data point is missing. The existing values are used if missing values exist in the data set. Pairwise deletion maintains more information than listwise deletion, which deletes absent cases.
Pairwise deletion is less biased for MCAR or MAR data, provided relevant mechanisms are covariates. Missing observations will degrade the analysis.
Missing data: Imputation
Imputation replaces missing values with an estimate. Use other data to form a comprehensive dataset.
You have numerous imputation options. The easiest way of imputation is to use the nasty or median of a variable.
Hot-deck imputation
Hot-deck imputation replaces missing values with values from related cases or participants. A “donor” value is used for each situation with missing values based on data from other variables.
Cold-deck imputation
In cold-deck imputation, missing values are substituted with existing values from similar cases in other datasets. The new values are derived from an independent sample.
FAQs
Missing values arise when you do not have data stored for particular variables or participants.
Missing data are important as they can influence results depending on the kind. Because of an unrepresentative sample, your results may not be generalizable.
Typically, you have the choice of accepting, eliminating or reconstructing missing data to organise it.