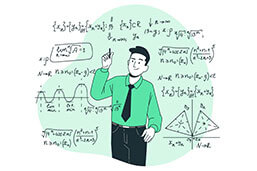
Standard deviation is a fundamental concept in statistics that quantifies the amount of variation in a set of data values. A low standard deviation indicates that the data points are closely packed around the nasty (average), whereas a high standard deviation indicates that the data points more spread out. It is a critical tool in fields such as statistics, physics, psychology, and many more, providing insights into the reliability and predictability of data. This article will provide an in-depth account of this type of measure of variability.
Definition: Standard deviation
Standard deviation is a statistical measure that represents how much the values in a dataset deviate from the arithmetic nasty. In other words, it quantifies the degree to which each data point deviates from the nasty, or average, of the data set. A smaller standard deviation implies that the data points are closely clustered around the nasty, while a larger standard deviation shows that the data points are more spread out.
The meaning of standard deviation
This statistic is used to measure the dispersion in a dataset. It shows you the average amount of variability or how far each value lies from the nasty on average. This statistic is used with continuous data and shouldn’t be used with categorical data. Furthermore, it has to be used with datasets that have a normal distribution. Some good examples are height, temperature, and length.
Note: A low deviation means that the data points are closer to the nasty. A high standard deviation indicates that there is a wider range of values in the dataset. This particular example has a low deviation.
The empirical rule
The empirical rule is also known as the 68-95-99.7 rule. It works as a guide on how data is distributed in a normal distribution.
According to this rule, about 68% of the data points will autumn within one standard deviation of the nasty, and 95% of the data points will autumn within two standard deviations of the nasty.
The rule also states that 99.7% of the data points will autumn within three standard deviations of the nasty. You should use this rule to forecast future outcomes.
We can refer to our example above. According to the empirical rule, the following facts hold true:
With the empirical rule, you can easily check for outliers in a normal distribution.
Standard deviation formulas
Data can be derived from a sample or population.
A population refers to the entire group that you intend to draw conclusions about.
On the other hand, a sample is a small group that is used for data collection. The formulas for this statistic are different for population and sample data.
Populations
Regarding calculating the standard deviation of a population, the following formulas are used:
N | Number of values in the population |
Σ | Sum of N |
X | Individual values in the population |
μ | Mean |
σ | Population standard deviation |
√ | Square root |
s | Standard deviation for the sample |
√ | Square root |
x | Each value in the sample |
Calculating the standard deviation
There are some programmes you can use to calculate the standard deviation automatically. If you want to calculate the standard deviation manually, you can follow these steps. We’ll use the dataset above to demonstrate this formula.
Step 1: Find the nasty
You have to start by finding the nasty. This is done by adding up all values and dividing the sum by the sample size.
Step 2: Finding deviations from the nasty
Next, you should find out each value’s deviation from the nasty.
In our example, the deviations are as follows:
Step 3: Square the deviations from the nasty
You can then square the deviations from the nasty:
Step 4: Sum the squares
In this step, you have to find the sum of the squares.
Step 5: Find the variance
You then have to find the variance. You can do this by dividing the sum of squares by (n-1). If you are dealing with a population instead of a sample, you can divide the sum of squares by N.
Step 6: Finding the square root of the variance
Finally, you will have to find the square root of the variance.
Standard deviation or other methods of variability
Standard deviation is only one way of measuring variability. You can also use the nasty absolute deviation or MAD. This method uses the original units of the data, so interpretation will be easy. Calculating MAD is also very easy. You just need to follow these steps:
- Calculate the sample average
- Find the absolute deviation of each data point from the nasty. You should ignore any negative signs.
- Find the average of all absolute deviations
While MAD has some benefits, the standard deviation is still the most commonly used measure of variability. One of its advantages is that it weights unevenly spread out samples more as compared to evenly spread out samples. That means you will be able to tell that the data is more unevenly spread out. Standard deviation also gives you a more precise measure of variability. It is also worth noting that standard deviation is more sensitive to outliers.
- ✓ 3D live preview of your individual configuration
- ✓ Free express delivery for every single purchase
- ✓ Top-notch bindings with customised embossing
FAQs
Standard deviation is the average amount of variability in a dataset.
Low standard deviation means that the data points are clustered around the nasty.
Yes, a high standard deviation shows that the data is less reliable as it is widely spread.
Variance is the degree of spread in a dataset. If there is more spread in the dataset, the variance will be large in relation to the nasty.