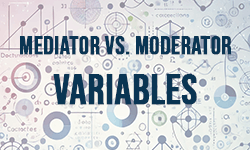
In the methodology of academic research, understanding the roles of mediators and moderators is crucial in exploring the relationships between different types of variables. While mediators explain why an effect occurs, moderators influence the strength or direction of these effects. In statistics, mediators and moderators help you understand the relationship between two variables. This article discusses the differences between mediator vs. moderator and a few examples.
Definition: Mediator vs. moderator
A mediator (mediating variable) explains the process in which two variables relate. In contrast, a moderator (moderating variable) affects the direction and strength of this relationship.
Mediator vs. moderator differ because of the following reasons:
- The mediator shows the connection between two variables. For instance, sleep quality (independent variable) affects the quality of your work (dependent variable) through alertness.
- The moderator may be acting upon two variables, changing the strength and direction of that relationship. For instance, mental health status can moderate the relationship between sleep and work quality. The relationship is stronger for people without mental health conditions than for their counterparts.
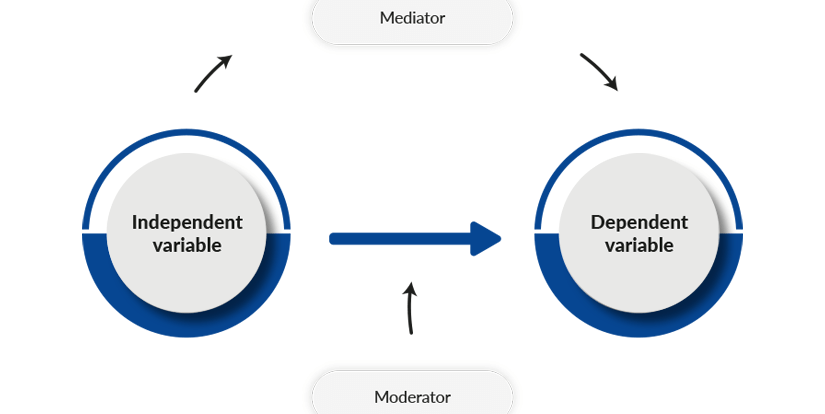
Mediator vs. moderator variables
An analysis of mediator vs. moderator variables is essential to understand the distinction between the two better, as explained below:
Mediation analysis
Mediation analysis tests whether a variable is a mediator using one of the two main methods – Analysis of Variance (ANOVA) or linear regression analysis. Mediation may either be partial or complete.
Taking the mediator out of the model in complete mediation eliminates the relationship between an independent and dependent variable. This is because the mediator thoroughly explains the relationship between a dependent and an independent variable.
In partial mediation, the relationship between the dependent and independent variable still exists when you take the mediator out of the model. This is because the mediator only partially explains this relationship.
When learning about moderating vs. mediating variables, understand that meeting the following conditions makes a mediation analysis feasible:
- The independent variable must cause the mediator
- The mediator must influence the dependent variable
- The mediator must cause a higher statistical correlation between dependent and independent variables
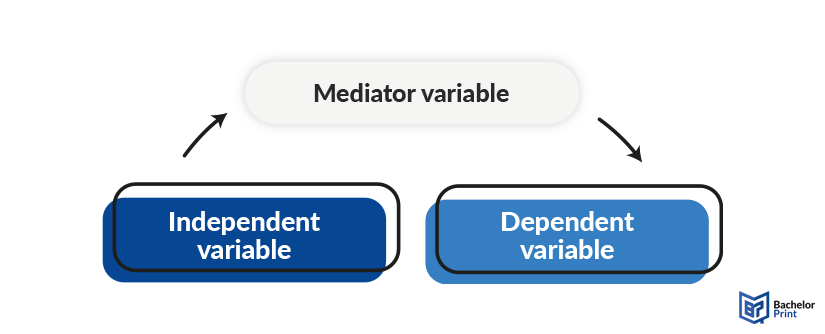
In simple linear regression, the models describe the connection between variables by fitting a line to the data you observe. Regression makes it possible to estimate how a dependent variable changes when the independent variable(s) change.
Simple linear regression is a parametric test estimating the relationship connecting two quantitative variables. In contrast, ANOVA is a statistical test that analyses the differences between the means of three or more groups. Both simple linear regression and ANOVA use the R program.
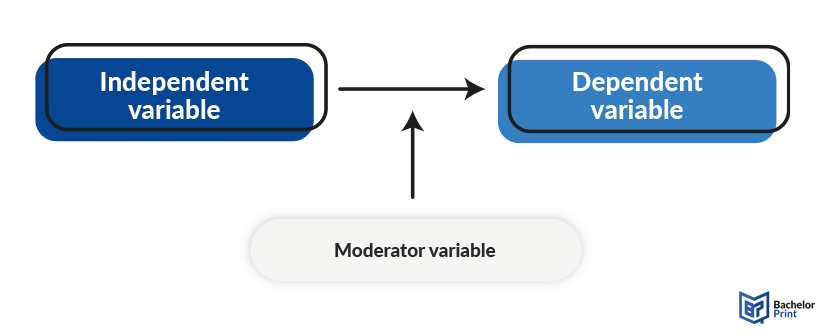
Moderation analysis
Moderation analysis tests the effects of a moderator variable on the relationship between a dependent and independent variable.
Multiple linear regression estimates the relationship between one dependent variable and two or more independent variables. You can perform multiple linear regression using the R program or conduct moderation analysis using Analysis of Moment Structures (AMOS).
Moderator variables are also called interactions or products. They may be qualitative (non-numeric values like education, gender, social status, etc.) or quantitative (numeric values like weight, age, test score, etc.) Moderator variables help judge your research’s external validity by identifying limitations when relationships hold.
It is important to know that in numerous instances, an influencing variable can be a mediator as well as a moderator. Often it is simply a matter of the phrasing of the research question. A mediator variable is always inquiring the “why?” of a relationship, while the moderator variable examines the “how much?”
Mediator vs. moderator examples
Here are some examples that identify the mediator vs. moderator variables as well as independent and dependent variables in research statements:
Example 1
A study on socio-economic status and reading ability in children:
Socio-economic status affects the children’s reading ability by influencing parental education levels. You hypothesize that parental education may influence children’s reading ability.
Independent Variable: Socio-economic status
Dependent Variable: Child reading ability
Mediator Variable: The parental education level influences the child’s reading ability.
Example 2
A study on salary and work experience:
You hypothesize that work experience years predict your salary while controlling relevant variables. Additionally, gender identity moderates the connection between salary and work experience.
Independent Variable: Work experience
Dependent Variable: Salary
Moderator Variable: The gender of the employee influences the salary.
Example 3
The influence of using a laptop at night:
You hypothesize that your mental health status may influence the hours you spend using your laptop at night, affecting your sleep hours.
Independent Variable: Using the laptop
Dependent Variable: Sleep hours
Mediator Variable: Mental health explains why people spend more or fewer hours on the laptop at night.
Example 4
The influence of social media on loneliness:
You hypothesize that social media may predict levels of loneliness; however, loneliness is much stronger for adolescents than adults.
Independent Variable: Social media use
Dependent Variable: Level of loneliness
Moderator Variable: The age of the person defines how likely social media influences their feeling of loneliness.
- ✓ 3D live preview of your individual configuration
- ✓ Free express delivery for every single purchase
- ✓ Top-notch bindings with customised embossing
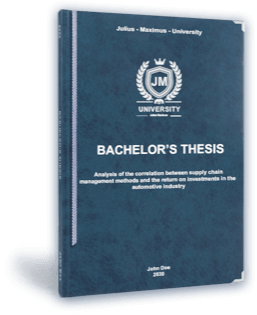
FAQs
At first, it appears that a mediator variable is just a confounding variable, but it is not. The difference is that a mediator variable itself cannot influence the dependent variable. A confounder, on the other hand, is related to the independent variable and can influence the dependent variable directly.
Mediators tell you why and how an effect happens, while moderators help judge the external validity of your research. Both variables are important in studying casual or complex correlational relationships.
A mediating variable is present when an independent variable does not directly influence the dependent variable but relies on an explaining variable in between that gives a higher statistical correlation between the dependent and independent variables.